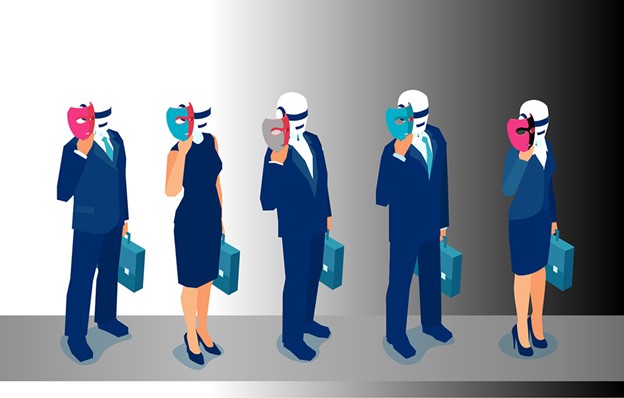
Mar
Responsible AI – What Causes Bias in Training Data?
jerry97890 comments artificial intelligence, Burning Questions
Over the past few decades, the use of AI in business has grown tremendously. By 2030, AI may generate up to $15.7 trillion in revenue to the global economy.
From process automation to sales forecast, the potential applications for AI in business are nearly limitless. But, you’ve still got to wonder, what happens when we place so much power on fairly new technology? Will it be fair or biased? Will it try to take over the world in the future? We don’t give the fear mongers much weight about AI taking over the world, but unchecked bias in AI data or models can lead to unintended negative consequences that do real harm.
That is where Responsible AI comes in. Responsible AI aims to address certain biases as well as create accountability for Artificial Intelligence systems. But, before we get to the benefits of Responsible AI, let’s check out the main issue it addresses; bias.
What is AI Bias?
AI bias is a form of output error in AI systems that results from prejudiced assumptions made during the training or development process. AI bias usually reflects common societal biases about gender, race, age, and culture. At its core, AI bias results from human prejudice, whether conscious or unconscious, that somehow manages to find its way into the training data.
Types of AI Bias
Bias in AI can come in multiple forms. Below are a few of the more common examples of how bias can influence AI-derived outcomes. In future posts, we’ll dig deeper into where and how bias can enter the AI process. Marketing leaders need to pay particular attention. Depending on how AI models are applied, at a minimum, could result in a PR nightmare. In a business context, relying on outcomes that are found to be biased could cripple your company by consuming resources, lead to defective products, or result in pursuing strategies that result in lost market share. While bias can lead to significant business issues, consequences can be even worse for humans and other living organisms. Situations such as misdiagnosed illnesses denied opportunities, and being misidentified in a manner that results in being incorrectly categorized can all have very serious consequences. Let’s look at some of the types of bias present in AI.
Selection Bias
Selection bias occurs when the training data is selected without proper randomization or is unrepresentative. Take this research, for example; three researchers compared the functionality of three commercial image recognition software to detect gender bias.
The study used AI tools to classify 1,270 pictures of parliamentary representatives in African and European countries. It was found that the tools performed better on male faces and showed a substantial bias against females with darker skin. On average, they failed over one in three women of color. All this could have been avoided if the training data had been more diverse.
Reporting Bias
Reporting bias arises from an inaccurate reflection of training data on reality. Take, for example, a reporting bias in a fraud detection tool that inaccurately marks all residents living in a certain geographical area as having a high fraud score.
In such a case, it is common to find that the training dataset the AI was given took every historical investigation in the area as a fraud case. This is very common in remote places where case investigators want to ensure that each case is indeed fraudulent before traveling to the area. Therefore, the number of fraudulent events in the training dataset is much higher than it actually is in reality.
Implicit Bias
Implicit bias occurs when an AI makes recommendations or decisions based on personal experiences that don’t necessarily apply generally. Say, for example, the AI picks up cues about women being housekeepers. In this case, the AI might find it difficult to connect women to more influential roles. A good example of this is the controversial Google images’ gender bias.
Group Attribution Bias
Do you remember when they told you not to hang out with the “bad crowd’ since you might get falsely accused of something? Well, group attribution bias works pretty much the same way.
Group attribution bias occurs when an AI attributes the qualities of an individual to a group the individual is or is not part of. This type of AI bias is mostly found in recruiting tools that tend to favor candidates from certain schools while showing prejudice against candidates who graduated elsewhere.
Why Do You Need Responsible AI in Your Business?
AI is a vital asset in the modern economy. It can promote corporate goals and boost business efficiency. But, for an AI to be efficient, it needs to properly incorporate a multitude of ethical and legal considerations.
You might think that eliminating bias is enough to avoid potential pitfalls, but in reality, it’s not. For that, you’ll need an algorithm that actively identifies ethical and legal risks that may result from its output. It should also act as a mitigating agent in these situations.
Responsible AI is guided by four key principles: fairness, transparency, accountability, and privacy & security. With these principles in mind, responsible AI can make more accurate decisions devoid of bias, thus resulting in a more efficient system.
Conclusion
AI has the potential to revolutionize the way you do business. Responsible AI addresses bias and incorporates ethical and legal considerations to provide you with accurate and efficient results. Contact Cascade Strategies for all your responsible AI needs.
Tags: advanced analytics, AI, ai in marketing, AI/ML, artificial intelligence, data driven marketing, market research analysis, product management, product marketing