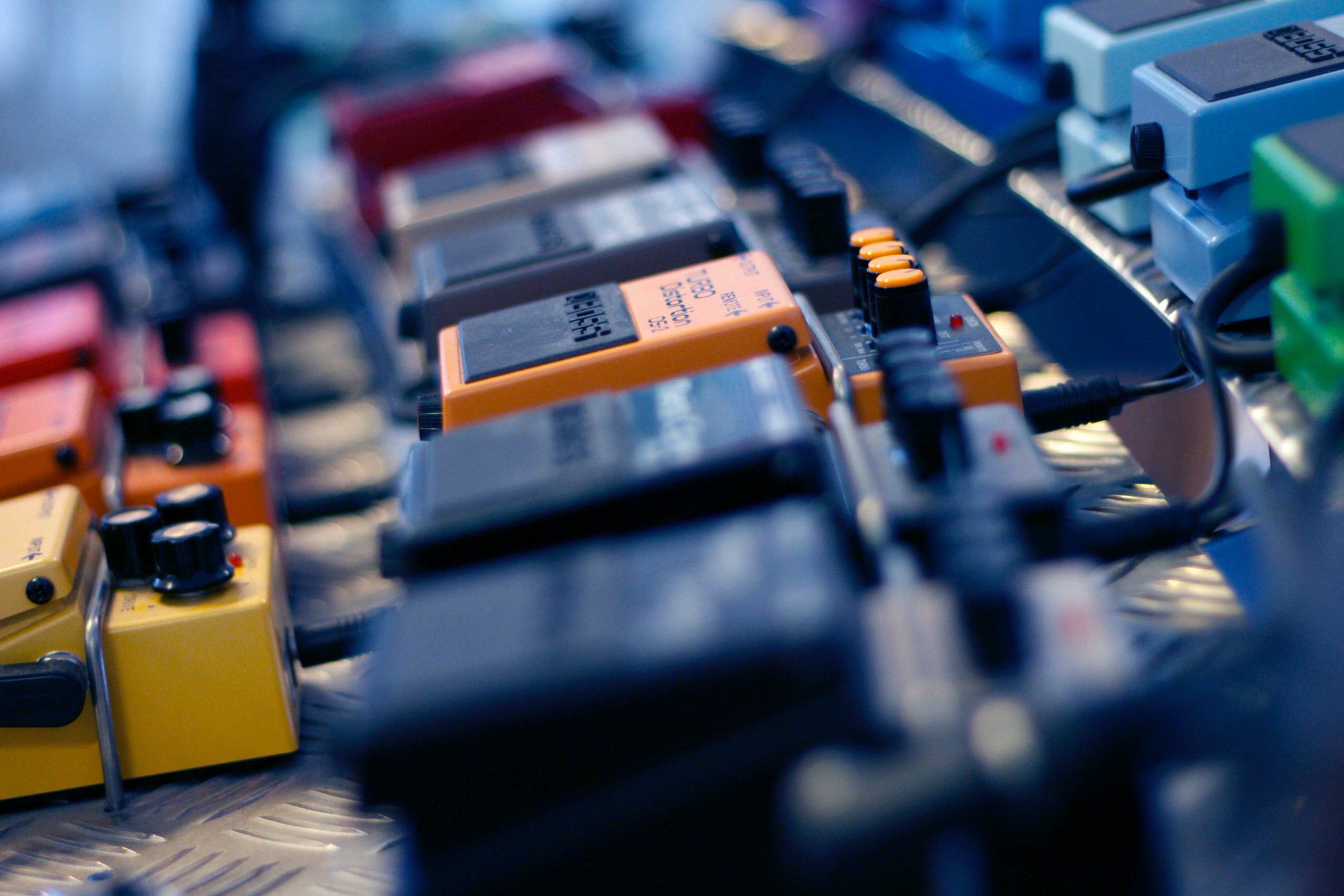
Nov
Applying AI: Healthcare Enrollment Optimization
Aleksey0 comments artificial intelligence, Burning Questions
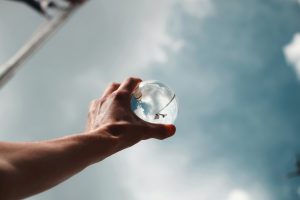
Proper application of AI techniques can yield clarity and precision of insights.
Here’s a case where AI rolled into the ongoing rollout of the Affordable Care Act (ACA). To avoid getting lost in the weeds, let’s just recall that there were big-money battles involving healthcare providers, the Federal government, and the confused, newly ACA-insured and wanted-to-be insured.
Problem: Two very large healthcare providers urgently needed to learn about the new members who came in through the ACA.
Like today, millions of Americans had millions in medical bills they were unable to pay. Big money was at stake for providers who would potentially have to take on those liabilities.
So who were the new ACA-driven guys? For their own readiness to serve, providers needed to know. What were their likely future care needs, their payment resources or shortcomings? What if they were uninsured and had no history?
One organization saw itself already losing millions. They called on an ACA provision that allowed them to sue the Federal government for repayment of losses. They needed accurate data to support their huge claims of loss.
The other organization tried a different approach: remediation, i.e., identifying high-risk customers and negotiating agreements to reduce likely losses.
But how to identify those customers? No human hands could possibly manage all of the data pouring in.
How AI machine learning managed a flood of data
Imagine a never-ending downpour. Trillions of raindrops, some useful, some toxic, each one interacting with others, changing as it falls depending on what it encounters.
Running this awesome show is our immune system, tasked with identifying each drop in a nanosecond and deciding its fate. Kill? Alter? Allow to pass untouched? Send elsewhere for further analysis?
Nice analogy, Mr. AI Guru! And so?
So the rain is trillions of bits of data. For data management, AI is our immune system.
And the machine learning (ML) model we devised paid off handsomely.
Insight 1: We were able to identify those few among the raindrops that were new recipients:
- Who were and would be active consumers of provider services and
- Who brought high net value to the provider, as defined by dollars paid in premiums minus the cost of providing those services.
But the learning didn’t stop at finding those profitable sweet-spot clients.
Insight 2: Among the millions of new clients eligible for remediation, the model found those whose cases promised the most immediate loss-mitigating results–and put them at the head of the queue.
Result? Millions saved.
Talk about intelligence–nothing artificial about that bottom line.
There’s a lot more where that came from. Service delivery strategies, product development, optimizing program results?
Yes, let’s talk about intelligence, none of it “artificial.” Give us a call at (425) 677-7430 or learn a bit more by visiting Cascade Strategies.
Tags: advanced analytics, AI, AI/ML, artificial intelligence, machine learning